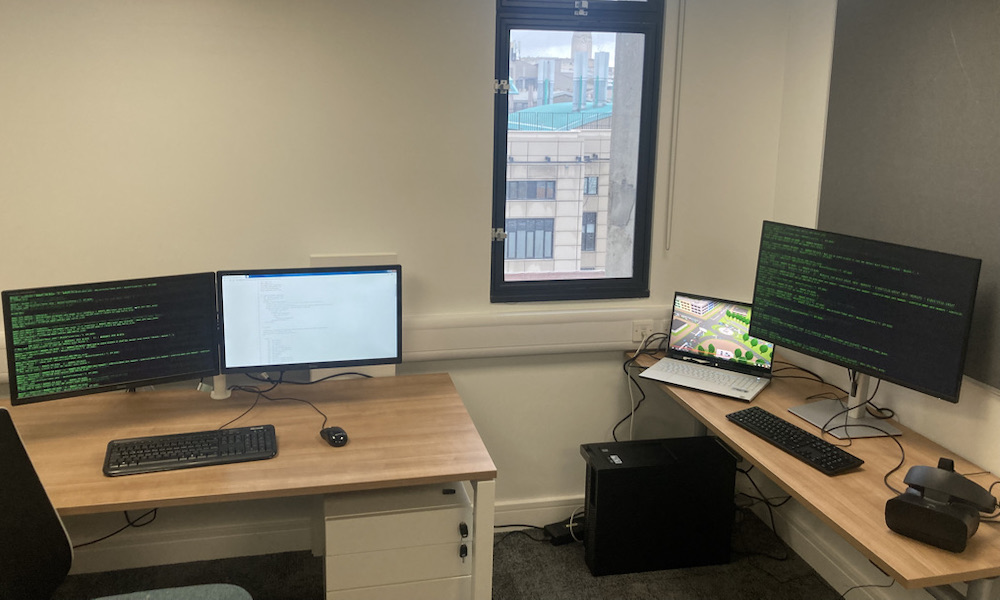
Case study for DAFNI site:
The University of Leeds: building digital twins of social systems
By Nik Lomax, Associate Professor of Data Analytics for Population Research at the University of Leeds and a Fellow at the Alan Turing Institute for data science and artificial intelligence. I am also co-Director of the ESRC-funded Consumer Data Research Centre (CDRC).
References: [1] [2] [3]
The use case
When applying to the DAFNI hardware fund, our use-case focused on building digital twins of social systems. Our research uses digital twin computer simulations, created with the hardware funded by DAFNI, to model social systems at a high resolution, both in terms of spatial scale and individual level attributes using techniques such as agent based models and microsimulation. Within a digital twin which represents the social system, we can investigate hypothetical policy change by running counterfactual experiments and assess how these impact on individuals, groups and spatial areas.
Our proposal included funding for two very high specification computers with powerful memory and processing power, so that we could carry out collaborative model building at speed. This now allows us to develop digital twin models across a variety of applications and to rerun them easily, and for the team to collaborate in a local environment rather than to use cloud based services such as Amazon Azure which come with additional running costs.
The high specification computation is essential for our work, but we also obtained further hardware for the creation and dissemination of results, including a large touchscreen monitor, projector and a VR headset to do some immersive work with these models. Two high specification laptops allow us to develop these models outside of the lab at Leeds. This hardware was also funded by DAFNI.
Building an augmented/ virtual reality world will help policymakers to make more informed decisions. The ability to take our research outside the university, to demonstrate the digital world to policymakers, means we will be able to better visualise scenarios and impacts.
How are you using the DAFNI equipment?
My research team create individual level models in a wide range of research contexts and are utilising the DAFNI hardware due to the requirement for high computational power to develop and run these models.
One strand of our work is the development of individual based microsimulation models for public health analysis. For example, in a project funded by the Turing, being undertaken in collaboration with the University of Southern California, we are building a dynamic model which we can use to assess the health and social status of the future elderly population in England. These models allow us to look at interventions on health conditions and modifiable conditions such as smoking and drinking.
As a second example, within the UK Prevention Research Partnership funded SIPHER (Systems science in Public Health and Health Economics Research) project we have created a digital twin representation of the UK population, drawing together census and survey data, which contains the attributes required for modelling health and social outcomes. Using dynamic modelling methods, we are assessing policy interventions and the associated pathways which would allow us to provide evidence on how best to tackle health inequalities. SIPHER consortium partners include The Scottish Government, Greater Manchester Combined Authority and Sheffield City Council. Our University of Leeds researchers are working with researchers at the universities of Sheffield, Manchester, Newcastle, Glasgow, Edinburgh and Strathclyde.
Another strand of our work focuses on infrastructure development. Individual level models are being built and run on the DAFNI hardware which produce high resolution population and household estimates and projections which are then used as input to infrastructure demand models. For example, we are currently collaborating with a transport consultancy, taking the output from one microsimulation model as the input to an agent based model and using this to assess different scenarios of transport infrastructure development.
The DAFNI hardware is located within the Leeds Institute for Data Analytics, and offers resources for a community of researchers within the institute. As a final example, the hardware has been utilised by researchers within the ESRC-funded Consumer Data Research Centre to assess physical activity patterns of individuals. This has involved clustering individuals based on their characteristics and activity profile to identify differences in behaviour. This is work that was made possible using the DAFNI hardware.
What does the DAFNI equipment allow you to do?
The DAFNI hardware allows us to develop collaborative models in a way we couldn’t previously. Before we installed the new hardware, solutions for model building with sufficient computational power required us to use paid for cloud-based services like Microsoft Azure, or to use the university’s High Performance Computing capability. The first of these attracted a monetary cost as these services work on a pay-per-use model while the latter could be expensive in terms of time because, as a shared service, we need to schedule jobs which can lead to delays. Having the DAFNI hardware gives us more flexibility in terms of time and money. Because we’re able to run models locally we have capacity to run more experiments. The hardware is allowing us to do research that wouldn’t have been able to do easily otherwise. For example, now we can implement computationally expensive machine learning and clustering algorithms – without good local compute power we would have had to use smaller datasets and not had such good outcomes.
We’re now able to carry out work that’s more experimental without fear of blowing the budget; and to allow researchers, especially PhD students, who may not have had access to compute because they are working on low budget projects, to move their project to a higher level.
How do you plan using DAFNI in the future?
Collaboration with stakeholders is going to be much easier once we’re out of the pandemic. When we applied for the hardware we had the vision that people would be in same room as each other, using tech such as VR headsets. Due to social distancing restrictions that has not yet transpired and we are not yet using interesting peripherals like that. However, once restrictions ease we will have more opportunity to do more with stakeholders – like local authority planners and analysts.
How does the university plan to link the hardware use with the DAFNI platform?
Now that we have the hardware that allows us to do the experimental collaborative modelling we can transfer models which have been developed locally on to DAFNI. This has the benefit of scalability if we need even more computational power. It also means we can take advantage of the exposure that DAFNI offers and increase the visibility and uptake of our models. We plan to put the models we’ve been developing onto the DAFNI platform in the future.
The hardware spec
Primary simulation machine:
- Dell Precision 7920 56 Core Intel Xeon Platinum Processor
- 1TB DDR4 ECC RAM
- Triple NVIDIA Quadro GPU (24GB)
- 1TB SSD HDD capacity
Secondary simulation machine:
- Dell Precision 5820 8 core Xeon Processor
- 128GB DDR4 ECC RAM
- 500GB SSD HDD capacity
Plus:
- Two Alienware Area laptops
- Oculus Rift and Touch
- Microsoft HoloLens 2
- Dell M318WL mobile projector
- 75” touchscreen monitor
Why use DAFNI?
The DAFNI platform offers both hardware and expertise. It offers a really good way to rapidly upscale and test out ideas. We already have an internal migration model on DAFNI, known as SIMIM, which forms part of a population forecasting model – and we were able to work with DAFNI tech team to make that model stable and accessible, and to run better on the platform than it does locally, which is really important.
Having SIMIM on DAFNI also gives us more exposure and allows more people to use the model. It provides a user interface for creating custom scenarios of infrastructure development at local level, which act as attractors of internal migrants and redistribute population. The resulting population changes can then be explored in an interactive web-based interface and alternative development scenarios can be compared.
As the platform is currently set up, you need to be reasonably tech savvy to engage with DAFNI, however lots of work is going on to make it more accessible. People developing models for their research are probably reasonably techhie anyway and it’s definitely worth using it if you have a need for compute power, want to increase collaboration in your models and have a need for visualisation of results.
[1] https://environment.leeds.ac.uk/geography/staff/1064/dr-nik-lomax