DAFNI Hardware at University of Sheffield
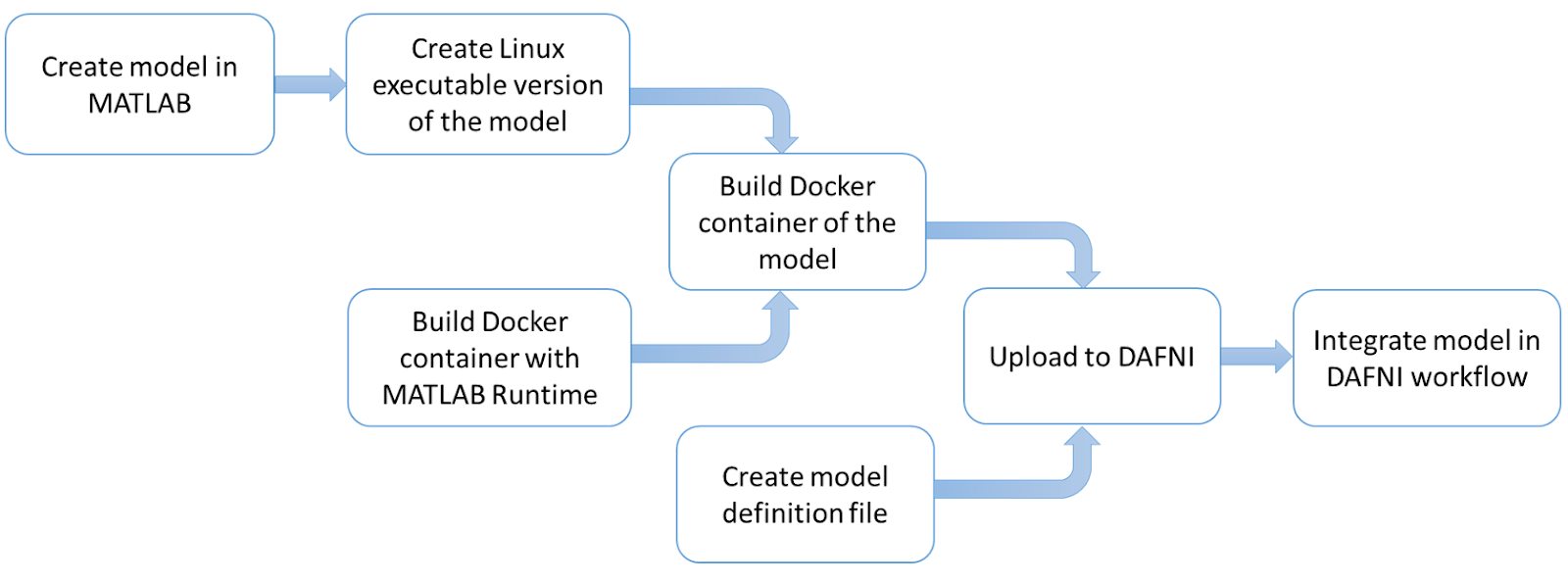
DAFNI hardware underpins University of Sheffield Traffic Digital Twin
By Professor Daniel Coca formerly, Honorary Professor of Nonlinear and Complex Systems, University of Sheffield. Professor Coca is now Head of School of Engineering and Professor of Nonlinear and Complex Systems at Newcastle University. The group continue their research in Sheffield using the DAFNI-funded equipment.
A grant from the Data & Analytics Facility for National Infrastructure (DAFNI) has allowed the Complex Systems and Signal Processing research group at University of Sheffield to purchase GPUs and other equipment to help it develop a Traffic Digital Twin. The high performance computing hardware is used to run simulations for large scale complex systems, solve computationally intensive multi-objective optimisation problems, as well as to train very large machine learning models that continuously learn from incoming data the traffic patterns to produce more precise traffic forecasts.
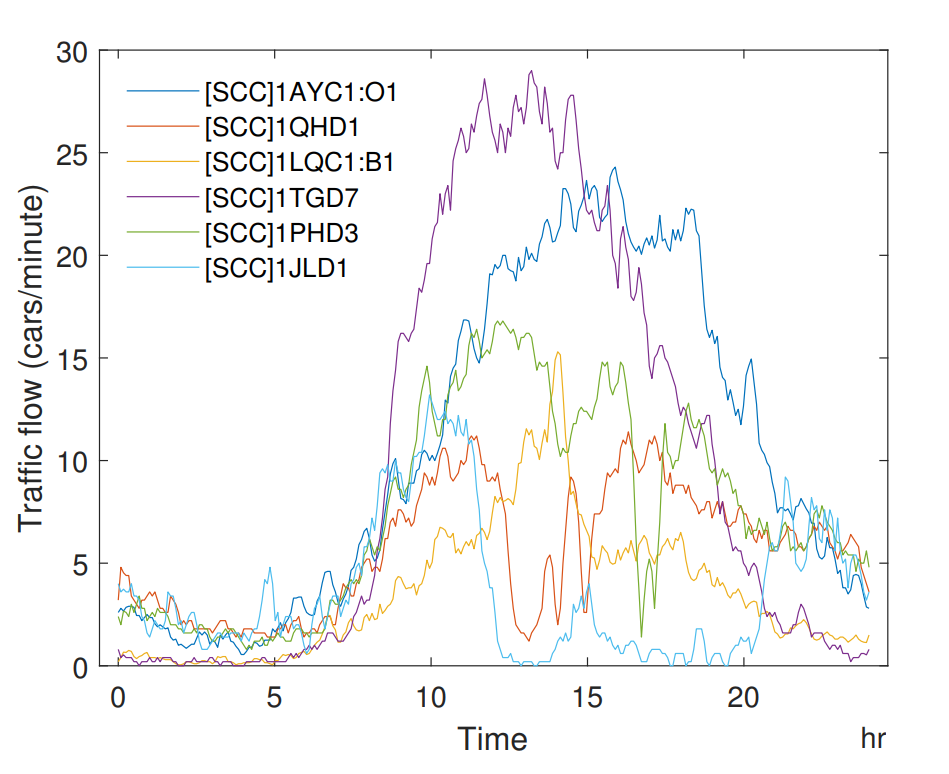
Research group
At Sheffield I led the Complex Systems and Signal Processing research group (CSSP). The group is renowned for its work on the identification and analysis of complex spatio-temporal systems, nonlinear signal processing, and the analysis and design of nonlinear systems in the frequency domain.
Many biological, chemical and physical systems involve variables which depend on both space and time. Such systems, which often operate away from equilibrium, exhibit complex emergent behaviour such as pattern formation, self organisation, turbulence, spatio-temporal chaos.
In general, this emergent behaviour cannot be predicted from the individual elements and their interactions. The only option to obtain more insight in these systems is by explicit simulation, which in general, is computationally expensive and the only feasible way is to apply massive parallel computing techniques to these models.
The group has pioneered the most advanced nonlinear system identification and information processing methodology, tools and algorithms available to date, which can be applied to a wide class of nonlinear complex dynamical systems, including chaotic, spatio-temporal and stochastic systems.
The tools developed as part of this research have been applied successfully to study heterogeneity in human embryonic stem cells, signal transduction in fly photoreceptors, iceberg calving patterns and climate change in Greenland as well as to model and analyse nitrous dioxide concentrations in urban areas and to develop a digital twin for motorway traffic.
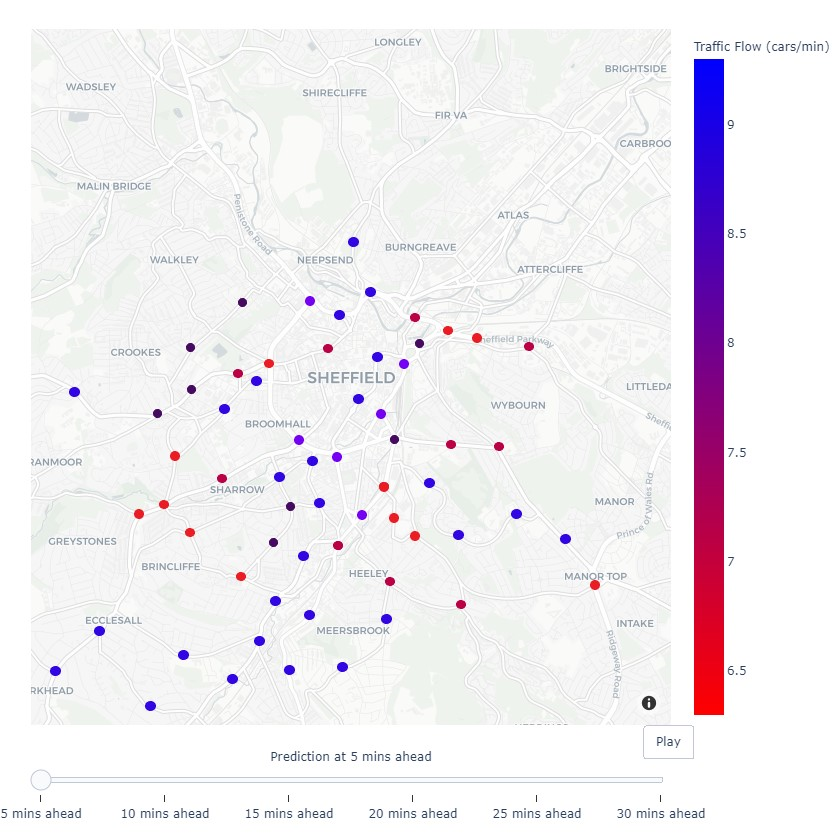
Use case for DAFNI hardware
Recent advances in internet of things technologies, data analytics, artificial intelligence, and cloud computing have enabled new possibilities for modelling in general, and infrastructure modelling in particular.
As the infrastructure systems are becoming increasingly cyber-physical and interconnected, there is a pressing need to develop next-generation systems engineering tools and methods for the design, development and operation of the future intelligent systems-of-systems.
The DAFNI hardware is being used to develop a Sheffield Traffic Digital Twin. The Digital Twin uses historic and real-time traffic data from more than 640 sensors in the Sheffield area to predict the evolution of the traffic and where congestion might occur.
The DAFNI hardware is being used to carry out computationally expensive simulations as well as to train very large machine learning models that continuously learn from incoming data the traffic patterns to produce more precise traffic forecasts.
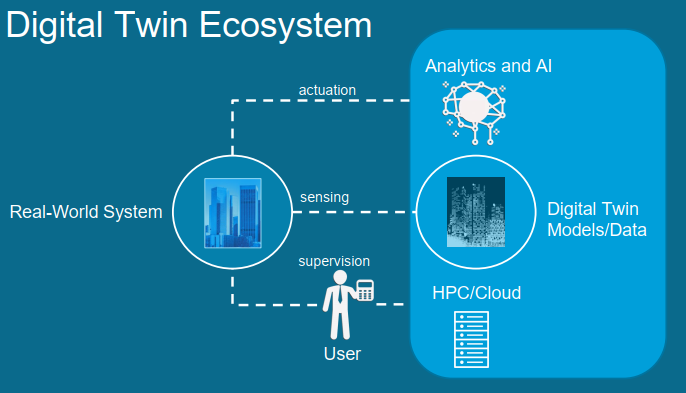
Could you describe the value the DAFNI hardware is adding to researchers
The DAFNI GPU hardware is used to run simulations for large scale complex systems, solve computationally intensive multi-objective optimisation problems, train large machine learning models of urban metabolism and evaluate the vulnerabilities of these models to adversarial attacks.
The hardware complements central DAFNI platform, helping to accelerate discovery and innovation in the area of infrastructure resilience and digital twins.
What difference is it making to researchers – what can they do now that they couldn’t do before?
The hardware is integrated within Sheffield’s HPC platform but access is managed, which ensures registered researchers have dedicated access to a local HPC-GPU facility, which enables them to run complex simulations and train large machine learning models faster.
How do you see yourselves using the DAFNI equipment in the future?
We envisage that the equipment will be used to build more advanced AI models that can power future digital twins for infrastructure operations.
How does your research/ the new DAFNI-funded hardware link with the DAFNI platform?
We are developing more advanced AI models that can learn urban traffic patterns, link based on data generated by urban observatories in Sheffield and Newcastle.
The hardware
2xC4140 server
Config K
Intel Xeon Gold 5120 2.2G, 14C/28T, 10.4GT/s , 19.25M Cache, Turbo, HT (105W) DDR4-2400
12x16GB RDIMM, 2666MT/s,
4 NVIDIA Tesla V100 SXM2 32G GPU Accelerator for NV Link