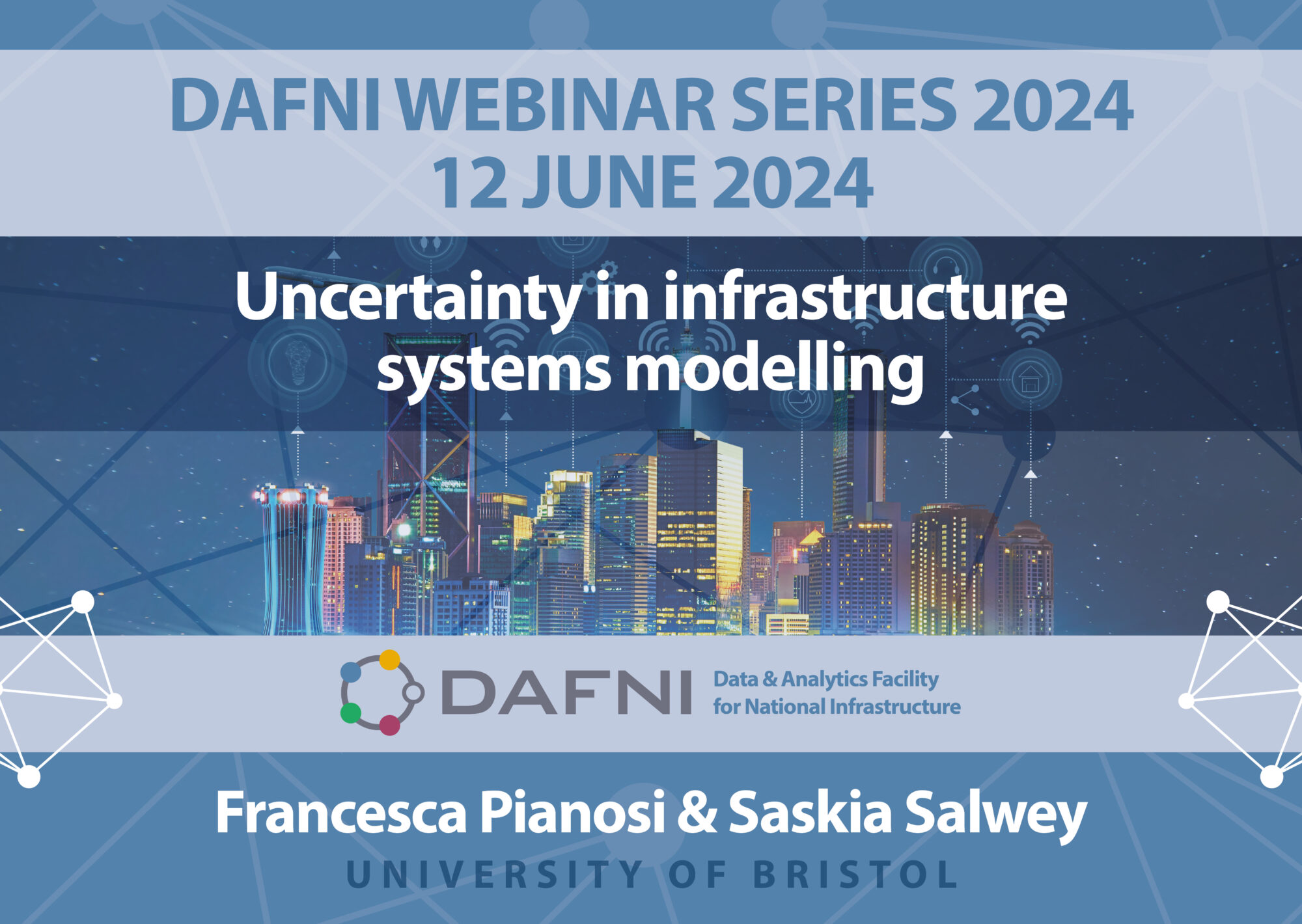
Handling uncertainty in infrastructure systems modelling
Webinar 12th June 2024
Francesca Pianosi, Associate Professor in Water and Environmental Engineering, and Saskia Salwey, Research Associate, both in the School of Civil, Aerospace and Design Engineering at the University of Bristol, are working together on the project with the DAFNI team on bringing uncertainty analysis into the DAFNI platform.
The USARIS project (Uncertainty and Sensitivity Analysis for Resilient Infrastructure Systems) will bring methodologies for Uncertainty and Sensitivity analysis of model outputs into DAFNI and deliver recommendations for best practice in their application. It will also investigate scalability and provide recommendations for future developments of the DAFNI platform to support the uptake of uncertainty and sensitivity analysis by the DAFNI users’ community.
They say:
Mathematical models are increasingly used to inform decisions in a variety of sectors from flooding to energy and from UK government decisions to EU policy level.
One of the challenges in using model outputs for policy decisions is that models are conditional on many uncertain assumptions. There is a lot of uncertainty around system drivers and properties, data gaps and errors, and simplifying assumptions.
For example, when using data for river flow rates in England and Wales where there is a relatively good measurement system, there can be an error margin of 30-50%. When trying to model using river flow rates in the Congo Basin where very little measuring is carried out, the margin of error will be considerably greater.
Models can also be used to look at investment decisions where scenarios involve long temporal horizons and, in this case, there is likely to be a lot of uncertainty around how the drivers of the system will evolve over time.
Another challenge is that when long modelling chains are built, it can cause a propagation of all the uncertainties that go into the model… and there is then a very large and broad range of uncertainties around what might happen.
Something to avoid is “spurious precision”, so it is important to identify the key sources of uncertainty in order to be able to hone those, to look at improving the model and its precision… and to understand when the model stops being valid.
With the USARIS project we are aiming to provide a generic methodology that can be used across models and sectors for quantifying the range of variability in the model outputs and the relative contribution of different uncertain inputs to that output uncertainty.
In the recent webinar (12 June 2024), we focused on ‘global’ Sensitivity Analysis – looking at varying all the inputs simultaneously and identifying where the uncertainty is mostly coming from.
Why are we doing Global Sensitivity Analysis?
This work will be useful for a range of different applications. For example, it can be used as a way to support the calibration of a model, which very often is a very complex and time-consuming task. Global Sensitivity Analysis aims to identify which are the parameters that mostly contribute to the accuracy of the model outputs, i.e. those which are more influential and therefore should be the focus of computationally expensive calibration. Out of 30 parameters you may find only 4 or 5 are affecting the model accuracy, once you have identified these using Global Sensitivity Analysis, future calibration work can focus on those parameters only.
Using Global Sensitivity Analysis you can gain some evidence to inform how you should prioritise efforts for reducing uncertainty – we can see where efforts for improving the model or quality of input data will have the most beneficial effect, and to help to target time and resources more accurately.
For example, if we take a model which aims to calculate the risk of losing days of work due to heat stress, it will involve a combination of many different components such as future temperature peak, a vulnerability component to show damage losses, an exposure map to highlight geographically where people or assets will be exposed to the hazard.
In this example we looked at various sources of uncertainty and tried to quantify the uncertainty in future risk and the key control of that uncertainty. We were able to identify future risk under different warming level scenarios and different hazard maps.
Using Global Sensitivity Analysis we could identify that the warming level is the most influential factor on risk but also that the second most influential uncertainty source is the vulnerability curve. The vulnerability curve is very often overlooked but we could show that this is much more important than the choice on how to bias-correct future climate predictions. This tells us that unless we can reduce the uncertainty in the vulnerability curve, we shouldn’t invest too much time on the other model components.
The importance of evaluating models
It is becoming more and more important to evaluate models, as they are increasingly used for policy decisions and health decisions, as we saw with the Covid modelling work which was heavily relied on during lockdown.
The evaluation is an important part of the USARIS work. For example, what are the dominant controls of the model outputs? Are the outputs sensitive to decision-relevant inputs? If we can’t establish that, then this is telling us that the model will tell us more about the consequence of the assumptions we make, than the difference between the policy options and scenarios.
The systems set up and configuration may be the most important control of the model predictions!
The USARIS tool is due to be available on DAFNI in Spring 2025.